Machine learning has always revolved around data – deriving patterns from the data, making predictions, taking decisions and performing actions without human intervention. Typically, connected devices on the edge are constrained by limited hardware capabilities and their role is restricted to gathering and transferring data from the edge. The task of processing this data and extracting insights takes place in the cloud.
This approach (collecting the data at the edge and computing it in the cloud) raises a number of challenges: latency, flexibility is compromised, and bandwidth requirements continue to increase as a result of the constant need to stay connected with the devices. Let’s take a closer look:
Bandwidth and Latency
Round trip delay resulting from API calls to the cloud (Remote server) can affect operations which require on-demand inferences.
For instance, security cameras working over sensitive regions like industrial centres, mining areas or border areas require on-demand inferences to trigger actions. Delay in action can affect the functionality of the system. In addition, the effective bandwidth is reduced when a large number of devices are employed.
Decentralization and Security
The security of generated and transferred data is of paramount importance to any organization. Systems with centralized data storage have a higher probability of DDOS attacks. Processing data at the edge devices helps to decentralize data storage, computing and less possibility of the entire system to be disrupted because of a single attack.
Why is ML computation moving from the Cloud to Edge?
RELATED BLOG
Over the years, devices on the edge have witnessed a rise in computational power. This has opened a door for edge devices to play a larger role within the entire IOT ecosystem, making it more responsive, intelligent and compact. Machine Learning and Artificial Intelligence can harness the unused computation power of the edge devices to enable a variety of solutions. An IDC study predicts that a whopping “45% of IoT-created data will be stored, processed, analyzed and acted upon close to, or at the edge, of the network – by 2019.”
How ML at the Edge Helps to Unleash the True Potential of IoT
Adding ML/AI to Edge devices can deliver a lot of benefits. Let’s take a look at some solutions that become possible with the combination of Edge Computing and Machine Learning.
Inferencing
Gesture recognition, image classification, object detection, acoustic analysis, and motion analysis can be performed on edge devices, thereby reducing the latency, bandwidth and security/privacy issues in the IoT systems.
Example: Security cameras, in addition to recording, can perform facial recognition. They can differentiate between a family member and an intruder to trigger specific actions. For instance, it can open the door for a family member and sound an alarm for an intruder.
Sensor Fusion
Affixing sensors for monitoring of each event increases the overall size and complexity of the IoT system. With the help of advanced machine learning and signal processing algorithms, these sensors can be combined to form synthetic sensors capable of processing complex events. This reduces the overall cost, enhances the quality of maintenance and improves energy efficiency.
Example: Autonomous driving technologies use Radar, Camera and LIDAR (Light Detection and Ranging Device) to estimate the location, velocity and trajectory of various classes/objects on the road. It is important to combine this information, correct inaccuracies from each device and generate a probabilistic state of the classes (objects) around it. One common algorithm used for this purpose is Kalman Filter.
Self-Improving products
Self-improving products are the future of IoT. Onsite devices can be programmed to undergo continuous improvements using the data collected, upload the information to the central server and update it with the combined result. This reduces the number of trials required to fully configure the devices, which makes implementation faster and more efficient.
Example: Google Android mobile keyboard Gboard uses Federated-learning technique to improve its suggestion rate from the information it has stored in the local devices. The trained prediction model in the cloud can be shared with the local devices, where it learns from the test data (data captured by the device via user interaction) and improves itself.
This newly trained model is uploaded to the cloud as a summarized update, using an encrypted communication channel to improve the overall shared model. The number of updates can be further reduced by compressing them using random notations and quantization.
Transfer Learning
Transfer learning, as the name suggests, utilizes the learning from the first task as a base for the second task related to it. This method has a vast scope of real world application, given the fact that enormous amount of data are required for training a learning model via deep learning methods.
Transfer learning is especially helpful when we do not have enough data to perform the training of the model. It uses the initial layer and middle layer as a base and training is performed on the latter layer leveraging the labelled data. The below diagram shows the benefits of this model.
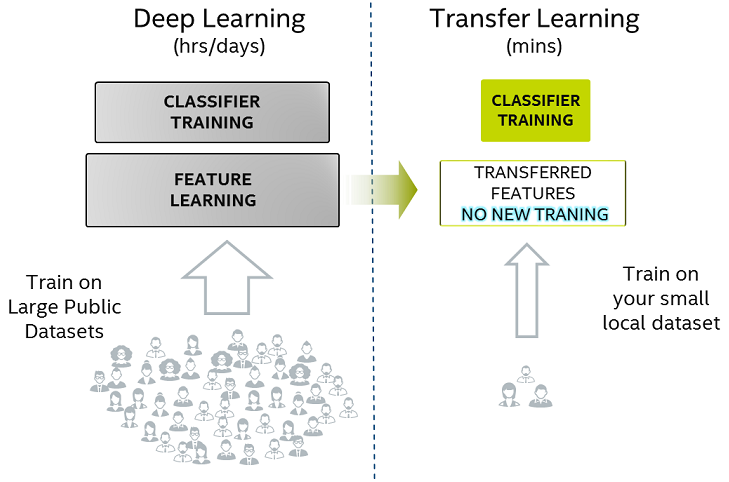
Partitioning the network layer and remodelling it offers flexibility to utilize the network for different applications, while working on the basis of previous models. Pre-trained models in the cloud can be shared over a number of local devices (Edge Devices) employing them for a number of tasks, with each device working in parallel. The result is a better functioning at the device level, plus overall performance improvement of the entire eco-system.
Example: For autonomous driving, vehicles can learn from the simulation data (pre-trained data) to differentiate activities on the road and take necessary actions in accordance to that. This promotes faster development and accuracy.
Technology pioneers are working on making the Edge Intelligent
NVIDIA launched Jetson TX-2 with Jetpack 3.0 to enable server-class AI computer performance on the edge devices. Amazon came up with AWS Greengrass to perform ML inferencing on the local devices. It allows building, training, and testing the model before deploying to the last segment of the IOT System. NVIDIA Jetson TX-1 and TX-2 and Intel Atom devices support AWS Greengrass.
Google developed a hardware chip Edge TPU with software stack Cloud IOT Edge. It allows to build, train and test models in the cloud. These models can be shared on the IOT Edge devices supported by Edge TPU hardware accelerator. Intel came up with its class of Xeon Processors designed for edge computing with low power design and high performance, which is well suited for Telecommunication service providers.
Microsoft and Qualcomm joined hands to develop devices with enough computation power to perform real-time inferencing along and with ample security features at the device level.
Vision AI developer kit for IoT, developed by eInfochips in partnership with Qualcomm and Microsoft, combines the edge computing power of the Qualcomm Vision Intelligence Platform from Qualcomm Technologies with Azure Machine Learning and Azure IoT Edge services from Microsoft.
Powered by Qualcomm Artificial Intelligence (AI) Engine, the kit enables on-device inferencing through integration with Azure Machine Learning and Azure IoT Edge. Use cases like visual defect detection, object recognition, object classification, motion detection, etc. can be easily developed and deployed in the Vision AI Developer Kit using models trained in Microsoft Azure, allowing uninterrupted 24×7 offline functioning.
The advanced camera processing, machine learning and computer vision software development kits adjoined with Qualcomm Snapdragon NPE (Neural Processing Engine) Framework provide analysis, development and debugging tools for different frameworks, inducing TensorFlow, Caffe and Caffe2.
It provides the much needed support for exploiting opportunities in the arena of security cameras, wearable cameras, virtual reality, robotics and smart displays.
ML at the Edge is the Way Forward
There’s no doubt that Machine Learning and Artificial Intelligence will make the Edge more intelligent and pave the way for next-gen solutions. AI in the edge is attracting developers and researchers to test their new ideas, develop models, customize them and reap benefits of AI in their work. eInfochips possesses expertise in both the areas – AI/Machine Learning Services and Cloud, IoT and mobility – and can help you bring intelligence to your edge devices. To know more, please get in touch with us.